Exploratory Data Analysis: Unraveling its Impact on Decision Making
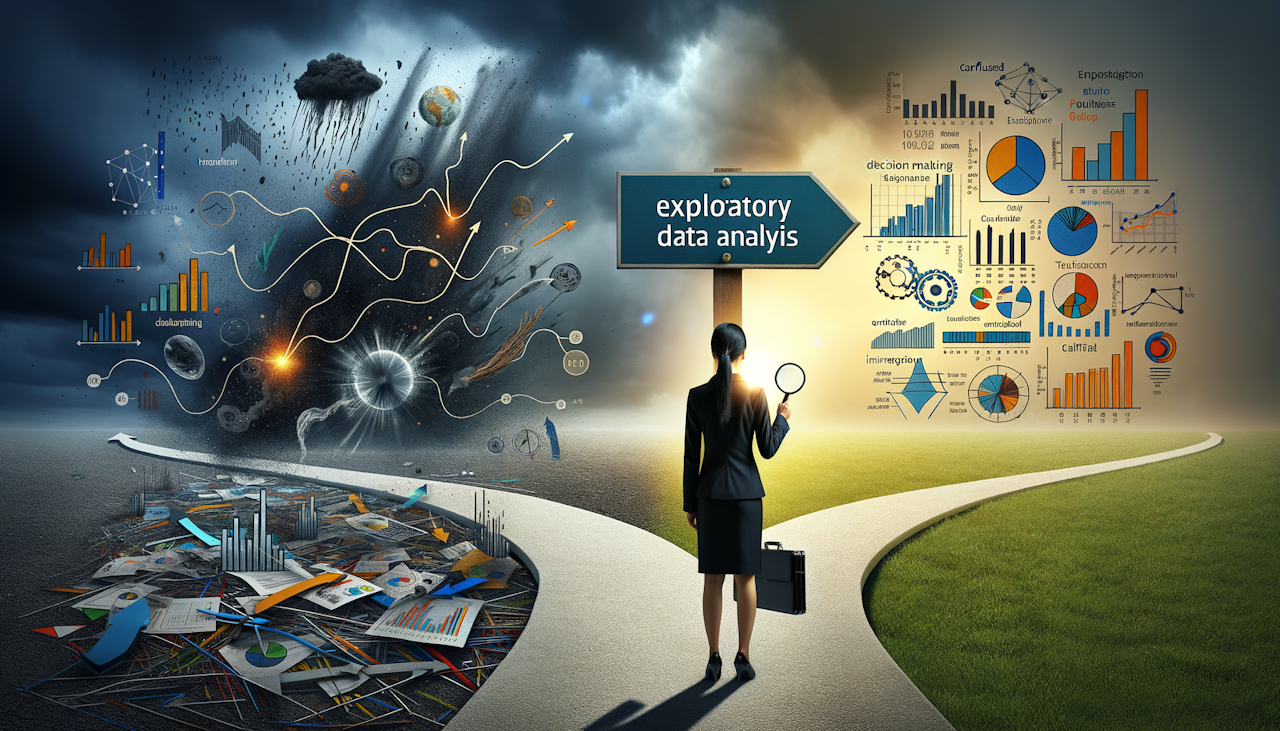
In an age where data reigns supreme, the pursuit of meaningful information stands at the forefront of decision-making across various industries. This article delves into the realm of Exploratory Data Analysis (EDA), an integral part of the data analytics process. Weaving together techniques that have been finely tuned over years of academic development and practical application, EDA reveals the narrative hidden within the numbers.
We will navigate through the conceptual underpinnings of EDA, its methodologies, their practical application in real-world scenarios, and the subsequent impact on decision-making. In pursuing this understanding, the article outlines not only the profound importance of EDA in contemporary analysis but reinforces its standing as a cornerstone of data science.
Introduction to Exploratory Data Analysis
At the very core, Exploratory Data Analysis is a philosophically grounded methodology aimed at discovering patterns, spotting anomalies, testing a hypothesis, and checking for assumptions. EDA is essential in making sense of data before formal modeling commences. It is this preliminary scrutiny that often dictates the viability and direction of subsequent analytical strategies.
The lineage of EDA can be traced back to the work of John Tukey, whose visionary perspective brought forth the concept in the 1960s. Tukey's approach to EDA was emblematic of a shift toward more robust, as well as intuitively guided, examination of data sets during the early stages of analysis. This philosophical expansion beyond mere number-crunching laid the groundwork for a more sophisticated and interpretable exploration of statistical information.
In the current narrative of data science and research, the role of EDA has only magnified. It is seen not merely as a preliminary step, but a fundamental practice within the entire lifecycle of data analysis. By virtue of this approach, researchers and analysts are better equipped to formulate more nuanced questions, design better studies, and craft more reliable predictions.
Major Goals of EDA
The primary objective within EDA is detecting anomalies and outliers, which are data points that deviate remarkably from the overall pattern of data. Identifying such anomalies is critical, as they can represent errors in data collection or novel insights that might significantly shift the study's trajectory.
Secondly, EDA is instrumental in testing underlying assumptions inherent in statistical models. This includes assumptions on the distribution of variables, linearity, and normality, which if unmet, can lead to erroneous conclusions. Therefore, EDA provides a safeguard against the risks associated with foundational analytical assumptions.
Lastly, EDA serves a crucial role by furnishing a concrete basis for further data analysis. It allows researchers to build a strong, informed foundation for the application of more formal and inferential statistical methods, ensuring that these techniques are applied appropriately and effectively with regard to the available data.
Techniques used in EDA
Exploratory Data Analysis is no monolith; rather, it comprises a vast array of techniques with unique advantages. These comprise numerical and graphical methods, each providing a lens through which to view and interpret the raw data available.
Numerical methods in EDA
Regarding numerical methods, EDA relies on a collection of summary statistics such as measures of central tendency (mean, median) and dispersion (variance, standard deviation). These metrics are the bedrock of data analysis as they provide a snapshot of the data's overall characteristic features.
When deploying these techniques, the insights can be revelatory. For instance, in analyzing customer satisfaction survey responses, the mean rating can reveal the overall satisfaction level, while the standard deviation can hint at variance in customer opinions. Numerically, one begins to shape an understanding of data distribution and identify where the focal points of intervention might lie.
Graphical methods in EDA
Conversely, graphical methods offer a visual entry point into data. Methods such as histograms, box plots, and scatterplots allow analysts to visually assess the distribution, detect patterns, and spot outliers. These methods translate the complexity of numerical data into more accessible and often more readily interpretable formats.
An example of this in practice could be the use of a scatterplot to identify the relationship between advertising spend and sales revenue. A visual association or trend can often yield insights more intuitively grasped than though raw numbers—allowing analysts to hypothesize about potential causation or decide on areas meriting deeper investigation.
Steps in conducting EDA
Applying EDA is not an arbitrary process, but rather one that benefits from a methodical and systematic approach. The sequence of steps one must follow can significantly influence the outcomes and interpretations derived from the analysis.
Data collection and preparation
A fundamental aspect of the analytical process is data collection and preparation, which sets the tone for the analysis. Gathering data in a methodical, rigorous way ensures its quality and reliability. Following collection, preprocessing is paramount—this process includes cleaning data, handling missing values, and ensuring that data types are properly assigned.
For example, before analyzing an online certificate course enrollment database, one must clean and preprocess the data to ensure that anomalies like duplicate records or incorrect data entries are rectified. Only then can the data be deemed reliable for further analysis.
Data characterization and Visualization
After the groundwork of preparation, EDA calls for data characterization and Visualization. This involves summarizing the data to understand its essential attributes and using various visualization tools to portray these characteristics. Both steps are critical in developing a coherent interpretation of the underlying data patterns and relationships.
Visualization tools give life to numbers, transforming abstract concepts into something visually graspable. Consider a course provider looking to analyze participant engagement in a problem solving course free of cost. By visualizing the data, it becomes possible to quickly discern time points or modules where engagement spikes or wanes—an invaluable insight for content improvement.
Analysis and Interpretation
The final steppes of EDA lie in the analysis and Interpretation of the data. This is where the actual exploration and pattern recognition takes place, followed by a thoughtful examination of these findings. The accurate modeling of data ensues, grounded in the insights gleaned through EDA.
Correctly interpreting the results is a skill as much as it is a science—taking the findings and distilling them into actionable insights. In this capacity, EDA is not merely an analytical procedure but a bridge connecting raw data to meaningful end-use applications.
The role of EDA in predictive modeling
The relevance of EDA stretches into the domain of predictive modeling, where it serves as a precursor to the application and refinement of statistical and machine-learning models.
Variable selection and transformation
In the context of predictive modeling, EDA helps in the critical task of variable selection and transformation. Through EDA, analysts can determine which features in the data are most relevant to the prediction task at hand and transform these variables to better fit the assumptions of the predictive models.
The benefit of this is clear: for example, through EDA, an analyst might find that log-transforming a skewed revenue variable results in a distribution that better suits the assumptions of a linear regression model, ultimately enhancing the model's performance.
Model validation
Model validation is another area where EDA proves indispensable. Before a predictive model is deployed, it must be rigorously tested—EDA assists in model validation by revealing patterns or anomalies in the residuals of a model, which might indicate model misfit or the need for additional feature engineering.
Consider a machine learning model predicting healthcare outcomes. Through EDA, one can assess whether the prediction errors are randomly distributed or if they display a pattern, hence determining whether systematic bias exists within the model.
The challenges and limitations of EDA
As powerful a tool as EDA may be, its application is not free of obstacles. The challenges and limitations of EDA necessitate a vigilant and informed approach to ensure its effective use.
One potential pitfall of EDA lies in the subjective nature of its process. Analysts must guard against the temptation to "over-interpret" visual patterns or outlier effects, which could skew the analysis. Moreover, EDA doesn't provide definitive answers but rather raises questions and hypotheses that must be addressed through further analysis.
To effectively tackle these challenges, one must cultivate a balanced approach, combining the insights from EDA with other quantitative methods and maintaining a critical eye on the analysis process.
Case scenario: EDA in action
A practical case scenario exhibiting the application of EDA is seen in analyzing customer behavior within a retail environment. Here, EDA can be used to segment customers based on their purchase history and identify distinct buying patterns.
Consider a situation where a retailer sifts through transactions to evaluate customer response to loyalty programs. EDA reveals that a particular segment of customers exhibits a higher frequency of purchases but lower average transaction value. This insight enables targeted marketing strategies to encourage higher spending within this group and fine-tune the loyalty program to better meet customers' preferences.
In conclusion, Exploratory Data Analysis reigns as an invaluable process in the refinement of decision-making within the realm of data science. This article illuminated the core principles, methodologies, and applications of EDA, highlighting its versatility and indispensable nature.
As we look toward the horizon, the future of EDA is expected to evolve in step with advancements in data collection methods, analytical tools, and computational power. Nonetheless, the foundational philosophies and practices outlined will remain critical waypoints in the journey towards data-driven knowledge.
Engagement with EDA requires not merely technical proficiency but also a spirit of intellectual curiosity. Those who seek to expand their analytical toolkit are encouraged to delve further into the subject, exploring the myriad resources available on EDA, including dedicated literature, practical workshops, and online certificate courses on offer in the expansive digital learning landscape.

He is a content producer who specializes in blog content. He has a master's degree in business administration and he lives in the Netherlands.